Team
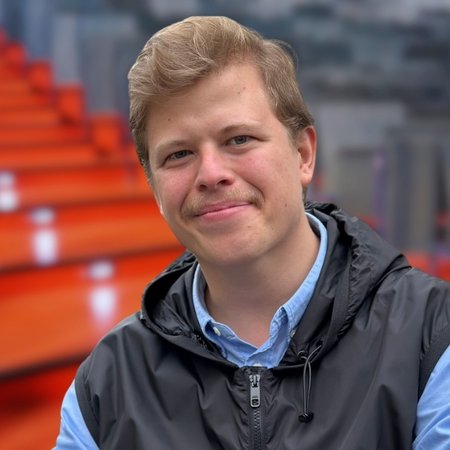
Academic Staff
Pierre Maier, M.Sc.
- Room:
- R09 R03 H40
- Phone:
- +49 201 18-34410
- Email:
- pierre.maier (at) uni-due.de
Bio:
If language shapes our reality and we have the capacity to change our language, it is possible to ameliorate our world through a purpose-driven change of our language. Information systems occupy a dual role in this regard. First, they must depict domain concepts, which thereupon influence their use within the domain – a reciprocal effect. Second, they can provide technical support for the generation and evaluation of alternative concepts. The application of appropriate software languages (modeling languages, programming languages) is essential for this purpose.
Multi-level software languages promise a higher degree of semantic expressiveness compared to traditional, two-level languages. Although they have been an active source of research for over twenty years, the adoption and dissemination of multi-level software languages is faced with severe challenges: (1) No standard multi-level language nor tool has yet been developed, (2) organizations often lack the required amount of expertise to use and develop multi-level languages, and, often times, (3) organizations already possess a huge number of two-level artifacts, which makes the transition to multi-level languages cumbersome and expensive. The realization of potential benefits of multi-level software languages calls for automated support in their creation and management. My research is centered around the investigation of automation techniques to support the automatic construction of multi-level domain models.
I recommend that bachelor and master students, who want to find a thesis topic in this field, consult literature of the following areas:
- Software-Language Engineering
- Language-Action Perspective
- Linguistic Relativity Principle
- Multi-Level Modeling
- Conceptual Engineering & Conceptual Ethics
- Generative Language Models
- Contingency and Possible Worlds
For literature advice or further questions conerning the formuluation of your thesis within the Information Systems discipline, I am happy to help. Furthermore, I offer to advise theses that can be broadly allocated in one of the following areas: artificial intelligence, machine learning, deep learning, data management, multi-level/enterprise modeling, business process management, organizational problem-solving, and philosophy of information.
I lecture in the following areas: Object-oriented modeling, (multi-perspective) enterprise modeling, data modeling, worfklow management systems, robotic process automation, integration with XML, integration with web services
Curriculum Vitae:
Professional Positions
since 04/2025: Research Assistant (Wissenschaftlicher Mitarbeiter) at the Research Group for Information Systems and Integrated Information Systems, University of Duisburg-Essen
since 08/2021: Research Assistant (Wissenschaftlicher Mitarbeiter) at the Research Group for Information Systems and Enterprise Modelling, University of Duisburg-Essen
10/2019 - 07/2021: Student Assistant (Wissenschaftliche Hilfskraft) at the Research Group for Information Systems and Enterprise Modelling, University of Duisburg-Essen
03/2018 - 01/2020: Working Student in the area of Metadata Managament, Data Governance, and Data License Management at E.ON Digital Technology GmbH, Essen
07/2017 - 12/2017: Working Student in the area of Database Testing at Finanz-Informatik GmbH & Ko. KG, Münster
Education
10/2015 - 04/2019: Business Information Systems, B. Sc., University of Duisburg-Essen
- Bachelor Project: "Design and Implementation of a Smart Contract-based Solution"
- Bachelor Thesis: "Design Thinking: Theoretical Background, Key Concepts, and Application Potentials in the Context of Software Development"
04/2019 - 05/2021: Business Information Systems, M. Sc., University of Duisburg-Essen
- Master Project: "Prototypical Development of an Accomodation App for Smart Urban Districts"
- Master Thesis: "Contingency Adaption Through Deep Learning: A Critical Reflection on the Applicability of Deep Learning for Organizational Problem-Solving"
Publications:
- Frank, Ulrich; Maier, Pierre: How an unintended Side Effect of a Research Project led to Boosting the Power of UML, 2025. doi:10.48550/arXiv.2505.09269) PDFCitationDetails
- Frank, Ulrich; Maier, Pierre: UML-MX©: Boosting Power of Object-Oriented Modeling and Enriching User Experience. In: Kaczmarek-Heß, Monika; Rosenthal, Kristina; Suchánek, Marek; da Silva, Miguel Mira; Proper, Henderik A.; Schnellmann, Marianne (Ed.): Enterprise Design, Operations, and Computing. EDOC 2024 Workshops: iRESEARCH, MIDas4CS, Doctoral Consortium, Joint CBI–EDOC Forum and Other Joint CBI-EDOC Events, Vienna, Austria, September 10–13, 2024, Revised Selected Papers. Springer, 2025, p. 276-286. doi:10.1007/978-3-031-79059-1_17CitationDetails
- Maier, Pierre; Schwarz, Tobias: UML++: Enhancing Student Learning of Object-Oriented Modeling through Executable Objects. In: MODELS Companion '24: Proceedings of the ACM/IEEE 27th International Conference on Model Driven Engineering Languages and Systems. 2024, p. 107-114. doi:10.1145/3652620.3687777CitationDetails
- Kühne, Thomas; Maier, Pierre: FMMLx and DLM: A Contribution to the MULTI Collaborative Comparison Challenge. In: MODELS Companion '24: Proceedings of the ACM/IEEE 27th International Conference on Model Driven Engineering Languages and Systems. 2024, p. 800-809. doi:10.1145/3652620.3688212CitationDetails
- Frank, Ulrich; Maier, Pierre; Töpel, Daniel: Modeling Facets of a Warehouse with the FMMLx: A Contribution to the MULTI Warehouse Challenge. In: ACM/IEEE International Conference on Model Driven Engineering Languages and Systems Companion (MODELS-C). 2023, p. 659-668. doi:10.1109/MODELS-C59198.2023.00107CitationDetails
- Frank, Ulrich; Maier, Pierre; Bock, Alexander: Low Code Platforms: Promises, Concepts and Prospects: A Comparative Study of Ten Systems - ICB Research Report, 70. 2021. doi:10.17185/duepublico/75244) CitationDetails
Tutored Theses:
- Comparative Analysis of Support Potentials from NLG Systems for Conceptual Modeling on the Example of UML Class Diagrams (Bachelor Thesis Business Information Systems, in progress)
- Reconciling Spreadsheet Editors and Multi-Level Modeling: Conception and Prototypical Implementation of Multi-Level-Model-Driven Spreadsheets Generation (Bachelor Thesis Business Information Systems, in progress)
- Smells and Anti Patterns in Software Programming and Modeling: Categorization and Outline of Solutions with Multi-Level Modeling on the Example of FMMLx (Bachelor Thesis Business Information Systems, in progress)
- LLM-Assisted Semantic Matching for Retail Category Management on the Example of GALERIA: Outline of a Process Model, Identification of Problem Areas, and Analysis of Support Potentials by Large Language Models (Bachelor Thesis Business Information Systems, in progress)
- Automatic (Meta) Classification for Bottom-Up Modeling: Challenges and Countermeasures (Bachelor Thesis Computer Science, in progress) AbstractDetails
Die Welt lässt sich als eine Menge interagierender Objekte betrachten. Diese Prämisse liegt der objektorientierten (OO) Programmierung zugrunde. Spätestens mit der Veröffentlichung von Smalltalk in den 1980er-Jahren hat sich die OO-Programmierung wachsender Beliebeit erfreut. Das hatte auch die Entwicklung von einer Vielzahl an OO-Modellierungssprachen und OO-Modellierungsmethoden zur Folge, die 1997 in die Standardisierung der UML mündete. Ein Kernkonzept der Objektorientierung ist dabei die sogennante “Klasse”. Eine Klasse dient als Schablone für Objekte; Objekte werden fachsprachlich von einer Klasse instanziiert. In gängigen Programmierungssprachen wie Java oder Python muss die Definition einer Klasse daher immer vor der Instanziierung von Objekten erfolgen. Dieser Schritt wird jedoch kritisiert: Für Menschen sei primär der Umgang mit Objekten natürlich - die Definition von Klassen stelle eine Herauforderung dar (vgl. Bergstein and Lieberherr 1991).
In den letzten Jahren hat in der konzeptuellen Modellierung die sog. “flexible Modellierung” oder auch “Bottom-Up-Modellierung” vermehrt an Resonanz erfahren. Auch hier wird die Notwendigkeit der strikten Top-Down-Modellierung kritisiert: Intuitiver sei es, Nutzern die Modellierung auf niedrigeren Ebenen zu ermöglichen. Der Bedarf nach Klassifikation von Objekten ist dabei nur einer von vielen Problembereichen.
Diese Bachelor-Thesis soll sich dem Problem der automatisierten Klassen-Induktion für die konzeptuelle Modellierung annähern. Technische Schwierigkeiten die bei der Programmierung aufkommen würden sind auszuklammern. Dabei kann entweder eine vergleichende Untersuchung existierender Ansätze vorgenommen werden oder es kann ein eigener Ansatz zur Klasseninduktion entwickelt werden. Für Letzteres könnten wir Ressourcen bereitstellen, auf denen aufgebaut werden kann. Details und genaue Themenausrichtung sind in Betreuungsgesprächen zu klären. In jedem Fall steht die Identifiaktion von Herausforderungen und eine Identifikation und Bewertung möglicher Gegenmaßnahmen im Kern der Thesis.
Literatur:
- Bergstein PL, Lieberherr KJ (1991) Incremental Class Dictionary Learning and Optimization. ECOOP '91 European Conference on Object-Oriented Programming: Geneva, Switzerland, July 15-19, 1991. Proceedings, pp 377–396
- Façanha RL, Cavalcanti MC (2014) On the Road to Bring Government Legacy Systems Data Schemas to Public Access. Proceedings of the 1st Joint Workshop ONTO.COM / ODISE on Ontologies in Conceptual Modeling and Information Systems Engineering co-located with 8th International Conference on Formal Ontology in Information Systems
- Guerra E, de Lara J (2018) On the Quest for Flexible Modelling. MODELS '18: 18th Intenational ACM/IEEE Conference on Model Driven Engineering Languages and Systems, pp 23–33
- Kessentini W, Alizadeh V (2022) Semi-Automated Metamodel/Model Co-Evolution: A Multi-Level Interactive Approach. Software and Systems Modeling 21:1853–1876
- Töpel D, Kaczmarek-Heß M (2022) Towards Flexible Creation of Multi-Level Models: Bottom-Up Change Support in the Modeling and Programming Environment XModeler. MODELS '22: Proceedings of the 25th International Conference on Model Driven Engineering Languages and Systems: Companion Proceedings, pp 404–413
- Towards a Method for Organizational Problem Solving with Large Language Models: Analyzing Prospects and Challenges (Master Thesis Business Information Systems, 2024)
- Natural Langauge Generation as a Complement to Conceptual Modeling? (Bachelor Thesis Business Information Systems, 2024) AbstractDetails
Natural languge processing (NLP) ist der Bereich der Informatik, der sich mit der algorithmischen Verarbeitung von natürlicher Sprache befasst. NLP lässt sich dabei in zwei Bereiche unterteilen: Das Verständnis von natürlicher Sprache (Natural Language Understanding, NLU) und die Generation von natürlicher Sprache (Natural Language Generation, NLG). NLG-Systeme sind zwar kein neues Phänomen, haben aber in den letzten Jahren durch Tools wie ChatGPT vermehrt an Resonanz erfahren.
Manche Autoren schätzen Modelle bzw. Diagramme teilweise als zu schwer verständlich ein, sodass für entsprechende Modellnutzer eine zu schwer überwindbare Hürde entsteht (vgl. Dalianis 1992, Gulla 1996). Natürliche Sprache in Form von kurzem Fließtext sei hingegen universell verständlich und soll daher dabei helfen können, diese Hürde zu überkommen (ebd.).
Je nach Interesse kann die Thesis unterschiedlich ausgelegt werden. Zum Beispiel kann untersucht werden, welche Ansprüche mit Modellen einhergehen (bspw. Beschreibung, Vorschreibung oder Erklärung von Situationen) und wie diese (besser?) durch NLG adressiert werden könnten. Alternativ kann auch ein prototypisches NLG-System entwickelt und evaluiert werden. Auch kann eine vergleichende Untersuchung angestrebt werden, wo die Potenziale für verschiedene Arten von konzeptuellen Modellen (bspw. GPML vs DSML) gegenüber gestellt werden.
Literatur:
- Ackermann L, Schönig S, Zeising M, Jablonski S (2015) Natural Language Generation for Declarative Process Models. Enterprise and Organizatonal Modeling and Simulation: 11th International Workshop, held at CAiSE 2015, pp 3–19
- Dalianis H (1992) A Method for Validating a Conceptual Model by Natural Language Discourse Generation. In: Loucopolous P (ed.) Advanced Information Systems Engineering, CAiSE 1992 Proceedings. Springer International Publishing: Cham, pp. 425-444
- Dalianis H, Johannesson P (1997) Explaining Conceptual Models: An Architecture and Design Principles. In: Embley DW, Goldstein RC (eds). Conceptual Modeling - ER '97 Proceedings. Springer: Berlin, Heidelberg, pp 215–228
- Gulla JA (1996) A General Explanation Component for Conceptual Modeling in CASE Environments. ACM Transactions on Information Systems 14(3):297-329
- Lavoie B, Rambow O, Reiter E (1997) Customizable Descriptions of Object-oriented Models. Proceedings of the Fifth Conference on Applied natural language processing -, pp 253–256
- Leopold H, Mendling J, Polyvyanyy (2012) Generating Natural Language Texts from Business Process Models. In: Ralyté J, Franch X, Brinkkemper S, Wrycza S (eds). Advanced Information Systems Engineering: 24th International Conference, CAiSE 2012 Proceedings. Springer-Verlag: Berlin, Heidelberg, pp 64–79
- Paris CL (1988) Tailoring Object Descriptions to a User's Level of Expertise. Computational Linguistics 14(3):64–78
- Reiter E (2007) An Architecture for Data-to-Text Systems. In: Busemann S (ed). ENLG '07: Proceedings of the Eleventh European Workshop on Natural Language Generation. Association for Computational Linguistics: Stroudsburg, PA, pp 97–104
- Reiter E (2010) Natural Language Generation. In: Clark A, Fox C, Lappin S (eds). The Handbook of Computational Linguistics and Natural Language Processing. Wiley-Blackwell: West Sussex, pp 574–598
- Sobieszek A, Price T (2022) Playing Games with AIs: The Limits of GPT-3 and Similar Large Language Models. Minds and Machines 32:341–364
- Machine Learning-assisted Domain Modeling: Survey, Classification, and Evaluation of Existing Approaches (Bachelor Thesis Business Information Systems, 2024) AbstractDetails
Conceptual modeling, although crucial to any enterprise, can be a troublesome and error-prone activity. The creation and maintenance of conceptual models can be time-consuming and low-quality models can negatively affect organziational decision-making. One root problem is that domain experts often lack respective modeling expertise and, vice versa, modeling experts often lack the the required domain expertice. Therefore, it is desirable to support conceptual modeling activities by computational means. The rise of machine learning has led to broad range of suggestions how this might be achieved. The suggestions thereby vary in scope and domain-specificty and, among others, conern the repairment or completion of conceptual models. I summarize these different ML-based approaches to support conceptual modeling under the name machine learning-assisted domain modeling, or MAD modeling for short.
This thesis should present a survey and classification of existing MAD modeling approaches. The core of thesis, or its main difficulty, is in the specification of an appropriate utility measure that supports an assessment of the diverse MAD modeling approaches.
Literature
- Almonte L, Guerra E, Cantador I, de Lara J (2022) Recommender Systems in Model-Driven Engineering: A Systematic Mapping Review. Software and Systems Modeling 21:249–280
- Barriga A, Rutle A, Heldal R (2022) AI-Powered Model Repair: An Experience Report — Lessons Learned, Challenges, and Opportunities. Software and Systems Modeling 21:1135–1157
- Hartmann T, Moawad A, Fouquet F, Le Traon Y (2019) The Next Evolution of MDE: A Seamless Intregration of Machine Learning into Domain Modeling. Software and Systems Modeling 18:1285–1304
- Saini R, Mussbacher G, Guo JLC, Kienzle J (2021) DoMoBOT: An AI-Empowered Bot for Automated and Interactive Domain Modelling. 2021 ACM/IEEE International Conference on Model Driven Engineering Languages and Systems Companion (MODELS-C), pp 595–599
- Yasdi R, Ziarko W (1988) An Expert System for Conceptual Schema Design: A Machine Learning Approach. International Journal of Man-Machine Studies 29(4):351–376
- Support Potentials of Language Models for the Construction of Conceptual Models (Bachelor Thesis Business Information Systems, 2024) AbstractDetails
Konzeptuelle Modellierung befasst sich im Kern mit Sprache: Modelle sollen sprachlich repräsentierte Konzepte erfassen und werden dafür mithilfe einer Modellierungssprache erstellt. Die Erstellung von konzeptuellen Modellen kann sich dabei verschiedenen Herausforderungen und Schwierigkeiten entgegensehen. Auf welcher Modellierungsebene soll ein Sprachkonzept abgebildet werden? Ist mein Modell hinreichend adaptiv für mögliche zukünftliche Veränderungen? Häufig ist dabei eine Diskrepanz festzustellen: Domänenexperten fehlt die erforderliche Modellierungskenntnis und Modellierungsexperten das erforderliche Domänenwissen.
Es ist daher wenig überraschend, dass das wachsende Interesse an sog. Sprachmodellen wie GPT-3 auch in Hinblick auf potenzielle Unterstützung für die konzeptuelle Modellierung hin untersucht wird. Sprachmodelle stellen eine bereits erlente Repräsentation von Komponenten der natürlichen Sprache bereit. Beispielsweise könnte hierdurch die Beziehung zwischen verschiedenen Domänenkonzepten erörtert werden.
Im Kern dieser Thesis soll sich mit der Frage beschäftigt werden, inwieweit Sprachmodelle Unterstützung bei der Erstellung von konzeptuellen Modellen anbieten können. Dabei können verschiedene Facetten der konzeptuellen Modellierung in den Fokus gestellt werden, wie zum Beispiel domänen-spezifische Modellierungssprachen (DMSLs), Mehrebenenmodellierung (MLM) oder es kann auch eine konkrete Modellierungssprache wie die UML in den Fokus gestellt werden. Die genaue Themenausrichtung sollte in den ersten Betreuungsgepsrächen genauer erarbeitet werden.
Literatur
- Biswas R, Sofronova R, Alam M, Heist N, Paulheim H, Sack H (2021) Do Judge an Entity by Its Name! Entity Typing Using Language Models. The Semantic Web: ESWC 2021 Satellite Events. Virtual Event, June 6–10, 2021, Revised Selected Papers, pp 65–70
- Butlin P (2021) Sharing Our Concepts with Machines. Erkenntnis
- Cámara J, Troya J, Burgueño L, Vallecillo A (2023) On the Assessment of Generative AI in Modeling Tasks: An Experience Report with ChatGPT and UML. Software and Systems Modeling 22(3):781–793
- Combemale B, Gray J, Rumpe B (2023) ChatGPT in Software Modeling. Software and Systems Modeling 22(3):777–779
- Fill H-G, Fettke P, Köpke J (2023) Conceptual Modeling and Large Language Models: Impressions From First Experiments With ChatGPT. Enterprise Modelling and Information Systems Architectures 18(3):1–15
- Rosenfeld R (2000) Two Decades of Statistical Language Modeling: Wehere Do We Go from Here? Proceedings of the IEEE 88(8):1270–1278
- Sobieszek A, Price T (2022) Playing Games with AIs: The Limits of GPT-3 and Similar Large Language Models. Minds and Machines 32:341–364
- Weyssow M, Sahraoui H, Syriani E (2022) Recommending Metamodel Concepts During Modeling Activities with Pre-Trained Language Models. Software and Systems Modeling 21:1071–1089
- Large Language Models as a Tool for Organizational Problem-Solving? (Bachelor Thesis Business Information Systems, 2024) AbstractDetails
So-genannte Large Language Models (LLMs; deutsch: “große Sprachmodelle”) haben in letzter Zeit vermehrt an Resonanz gewonnen. OpenAIs ChatGPT und GPT-3 oder Googles BERT und LAMBDA sind nur einige damit in Verbindung stehende Beispiele. Häufig scheinen die ersichtlichen “Fähigkeiten” dieser LLMs für große Aufruhr zu sorgen. So werden sie unter anderem sogar als Ko-Autoren in wissenschaftlichen Publikationen angegeben (Stoel-Walker 2023).
Sprachmodelle erlernen eine statistische Repräsentationen von verschiedenen Sprachkomponenten wie Wörtern (vgl. Luitse und Denkena 2021, S. 1). LLMs, neben technischen Besonderheiten, stellen eine bereits erlernte Repräsentation von einer oder mehrerer Sprachen bereit. Der Clue dabei: Sprache ist kein Selbstzweck, sondern soll Informationen und Wissen verkörpern (vgl. Manning 2022, Petroni et al. 2019). Die Einsatzpotenziale von LLMs sind kaum genau erforscht – vor allem was mögliche Grenzen für die organisationale Praxis angeht. Diese Thesis soll LLMs kritisch mit Hinblick auf ihre Unterstützungsmöglichkeit für organisationales Problemlösen untersuchen. Falls gewünscht kann die Thesis auf konkrete Bereiche der Problemlösung (bspw. nur Problemformulierung oder nur bestimmte Problemkategorien) fokussiert werden. Solche interessenbasierte Anpassungen können wir dann in Betreuungsgesprächen klären.
Für BA-Thesis: Eine Bachelorarbeit zu diesem Thema sollte die Frage erörtern für welche Bereiche des organisationalen Problemlösens, LLMs anwendbar sind bzw. angewendet werden sollten.
Für MA-Thesis: Eine Masterarbeit sollte auf den Anforderungen einer BA-Thesis aufbauen und bspw. eine Methode entwerfen, welche den Einsatz von LLMs in Organisationen unterstützt. Das betrifft spätestens hier auch die Reflektionen über entsprechende Prompts.
Literatur:
- Getzels JW (1982) The Problem of the Problem. In: Hogarth RM (ed) Question Framing and Response Consistency. Jossey-Bass: San Francisco, Washington, London, pp 37–50
- Landry M (1995) A Note on the Concept of “Problem”. Organization Studies 16(2): 315-343
- Luitse D, Denkena W (2021) The Great Transformer: Examining the Role of Large Language Models in the Political Economy of AI. Big Data & Society 8(2):1-14
- Manning CD (2022) Human Language Understanding & Reasoning. Daedalus 151(2):127–138
- Newell A, Simon HA (1972) Human Problem Solving. Prentice-Hall: Englewood Cliffs, NJ
- Preston AM (1991) The “Problem” in and of Management Information Systems. Accounting, Management & Information Technology 1(1):43-69
- Petroni F, Rocktäschel T, Lewis P, Bakhtin A, Wu Y, Miller AH, Riedel S (2019) Language Models as Knowledge Bases? Proceedings of the 2019 Conference on Empirical Methods in Natural Language Processing and the 9th International Joint Conference on Natural Language Processing, pp 2463–2473
- Stoel-Walker C (2023) ChatGPT Listed as Author on Research Papers: Many Scientists Disapprove. Nature 613:620–621
- Data Provenance/Data Lineage: Reconstruction of Core Concepts and Analysis of Prospects and Challenges for Enterprise Modeling (Bachelor Thesis Business Information Systems, 2024)
- Project Leadership Enablement in Agile Project Management: Creation of a Process Model and Evaluation on a medium-size IT company (Master Thesis Business Information Systems, 2023)
- Metadata-enhanced Data Warehousing: Conceptions and Contributions to Data Integration (Bachelor Thesis Business Information Systems, 2023)
- XAI Approaches from the Perspective of Legal Requirements: Design and Use of an Evaluation Frame (Bachelor Thesis Business Information Systems, 2022)
- Business Process Automation through Robotic Process Automation? Critical Analysis and Synthesis of existing Criteria Catalogs for the Identification and Selection of eligible Business Processes (Bachelor Thesis Business Information Systems, 2022)
- Data Governance: Reconstruction of key concepts and analysis of implementation using existing enterprise modeling approaches (Bachelor Thesis Business Information Systems, 2022)
- Convolutional Neural Networks: Technical Foundations and Critical Evaluation of their Use in Organisations (Bachelor Thesis Business Information Systems, 2022)
- Action-theoretic Perspective: A Beneficial Extension for Enterprise Modeling? (Bachelor Thesis Business Information Systems, 2022)
- In-Memory Databases: Technical and Conceptual Foundations and Comparison of Selected Systems (Bachelor Thesis Business Information Systems, 2022)
- Use of Machine Learning in the Manufacturing Industry: Design of a Method for the Identification and Selection of Application Possibilities (Master Thesis Business Information Systems, 2022)
- Customer Churn Prediction in Literature and Practice - Design of a Process Model for the Selection of a Churn Prediction Model in Contractual Settings (Bachelor Thesis Business Information Systems, 2022)
- Problem structuring methods in requirements engineering – Development of a suitability framework for use in requirements elicitation (Bachelor Thesis Business Administration, 2022)
- Deep Learning in Organizations: Development and Application of an Evaluation Framework for the Comparison of Explainable Artificial Intelligence Methods (Bachelor Thesis Business Information Systems, 2022)